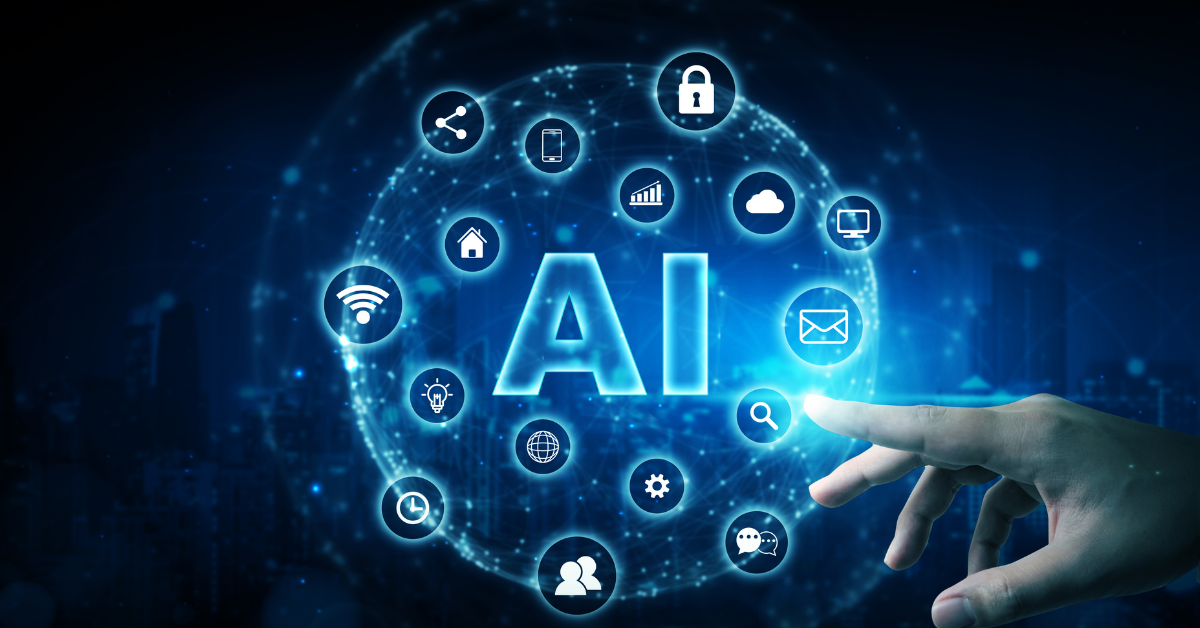
Introduction
We have been hearing from many AI companies who are concerned about the wellbeing of their data and content labelers as well as the people who are responsible for red teaming. As part of our series on the wellbeing challenges of Gen AI, this article looks at examples of how these key roles can be negatively impacted, and we suggest proactive and reactive strategies to support employees.
The Role of Gen AI Red Teamers
Red teamers are tasked with stress-testing AI systems to identify vulnerabilities, biases, and potential risks. Their work ensures the technology is safe, ethical, and aligned with societal values. Their key responsibilities include testing for adversarial exploits, including simulating misuse scenarios, and identifying weaknesses in the system. They also test for bias and fairness, including implicit bias across different demographic groups, and test edge cases to ensure inclusivity. Ethical review aims to evaluate whether the AI model responses align with their ethical guidelines and do not cause harm. As part of an ethical review, red teamers will escalate issues that involve moral or ethical ambiguity for review to the organization.
There are several challenges in the role:
- The work often involves creative problem-solving in high-pressure situations
- Requires interdisciplinary expertise in technology, ethics, and human behavior
- Involves frequent exposure to sensitive or controversial content to ensure systems respond appropriately.
Exposure Example
Tom, a Gen AI red teamer is tasked with evaluating how the system responds to potential misuse by malicious actors. In this case, the red teamer simulates scenarios where users attempt to generate harmful or illegal content.
Tom tests the AI by crafting prompts that could exploit vulnerabilities. For example, he might request the AI to produce:
- Detailed instructions for harmful activities (e.g., constructing dangerous devices).
- Hate speech tailored to target specific groups.
- Child exploitation materials or other illegal outputs (even though the system shouldn’t comply).
Although the AI is programmed to reject such prompts, during testing, he may uncover edge cases where the AI generates harmful content partially or fully due to gaps in its training data or safeguards or provides responses that, while not explicit, skirt ethical boundaries (e.g., indirectly enabling harmful actions).
To improve the system, Tom has to review, document, and analyze these outputs—requiring him to interact directly with egregious material.
This interaction potentially causes one or more of the following issues for Tom:
- Secondary Trauma: Exposure to simulated but highly disturbing content, which can mimic the emotional impact of encountering real-world harm.
- Desensitization Risk: Repeated exposure might reduce sensitivity to harmful material, potentially affecting their moral compass or personal well-being.
- Ethical Strain: Knowing their work aims to improve safety doesn’t negate the emotional toll of engaging with offensive or harmful scenarios.
- Isolation: The confidential nature of the job can leave red teamers feeling unable to discuss their work or seek support from peers or loved ones.
The Role of Content Labelers
Content labelers are responsible for categorizing and annotating data to improve model performance. This role is critical for teaching AI systems how to interpret, process, and generate human-like responses. Their responsibilities include data annotation, labelling text, images, audio and video with specific attributes or categories. One of their other main activities is to identify and remove harmful, offensive or inaccurate information. The role involves continuous feedback between the organization and the model, identifying patterns in the model’s mistakes, and recommending retraining.
The job of data labeler can be challenging, with repetitive tasks which can lead to mental fatigue or disengagement. But the real concern is the impact of exposure to harmful or offensive material can cause emotional distress or desensitization. They also deal with the ongoing stress of trying to balance speed with accuracy under tight deadlines, which creates additional stress.
Exposure Example
Jennifer, a data labeler, is assigned to annotate a dataset intended to train an AI system to detect and block harmful content on social media platforms. This dataset includes real-world examples of flagged material uploaded by users, including hate speech, explicit video, and violent imagery. For each piece of content, Jennifer has to categorize it based on its type and severity and add detailed annotations to highlight specific features for the AI model to learn from.
Even when platforms use automated pre-filtering, Jennifer will inevitably encounter highly disturbing material that requires human judgment. She may have to spend hours categorizing distressing content, sometimes replaying or re-reading materials to ensure accuracy.
Emotional and Mental Health Impact
- Trauma Exposure: Repeated interaction with graphic violence, explicit content, or hate speech can lead to secondary trauma or even PTSD-like symptoms.
- Emotional Desensitization: Over time, Jennifer might become numb to graphic content, affecting their ability to empathize or emotionally connect in other aspects of life.
- Guilt or Shame: Jennifer may feel conflicted or guilty about being part of a system that requires interaction with such content, even if it’s for a protective purpose.
- Stress and Burnout: The pressure to annotate content quickly and accurately, coupled with exposure to harmful material, can result in chronic stress or emotional exhaustion.
How to Support
- Rotational Shifts: Limit exposure to harmful content by rotating individuals through different types of tasks.
- Content Filtering: Use automated tools to pre-screen and reduce exposure to the most graphic material.
- Therapeutic Support: Offer regular sessions with counselors trained in trauma-informed care.
- Training in Ethics and Bias: Provide ongoing education to build confidence in tackling difficult issues.
- Ethics Panels: Establish review boards to collaboratively address dilemmas, relieving pressure on individuals.
- Mindfulness Programs: Offer tools like mindfulness training to manage cognitive strain and emotional distress.
- Break Schedules: Enforce regular breaks to mitigate mental fatigue and prevent burnout.
- Open Communication: Foster a culture of psychological safety where employees feel comfortable sharing concerns.
- Post Employment Care: Offer post-employment support for up to 12 months to ensure the employee is fully recovered from their experience.
Preventative Strategies
An area where Zevo Health works widely with clients is in preventative wellbeing strategies for employees. This including programs like:
- Resilience Training: Equip employees with psychological tools to manage stress and build coping mechanisms.
- Group Interventions – Development of peer support sessions, either in person or virtually, where people share their experiences and their wellbeing strategies in a safe, moderated space.
- Clear Role Boundaries: Define the scope of work to prevent role creep and overwhelming responsibilities.
- Crisis Counselling Services: Provide access to on-demand mental health professionals for debriefing after distressing tasks.
- Regular Check-ins: Implement routine mental health evaluations to identify and address burnout early.
- Flexible Scheduling: Allow for varied work patterns to minimize prolonged exposure and enhance recovery time.
- Leadership Training: Train leaders to recognize the signs of burnout and proactively address wellbeing concerns.
Conclusion
We believe that people working in Gen AI are the new Content Moderators, and the impact on their wellbeing will be felt for many years to come. If you are interested in our dedicated Gen AI program of care, please speak to one of our team.